Erosions on X-ray: can we delegate this to the computers? Save
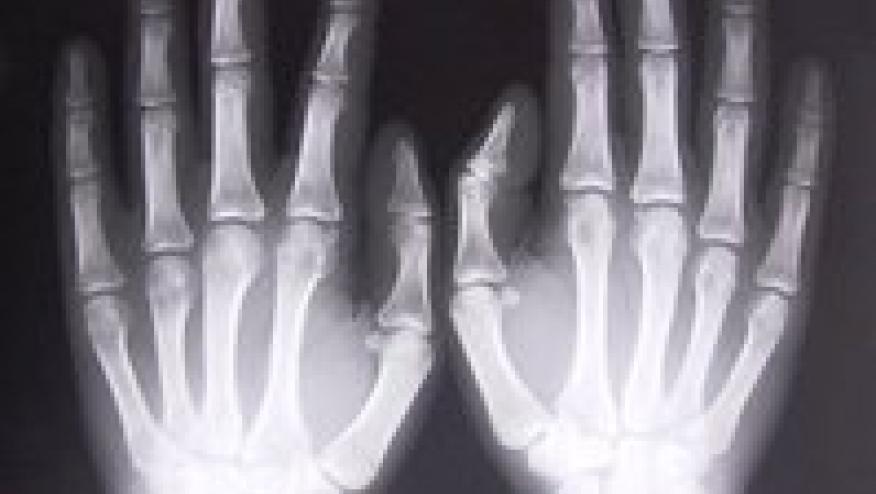
The prospect of automating hand x-ray scoring reliably has always been an enticing one, and data presented at the American College of Rheumatology (ACR) Convergence 2020 suggest this might be in reach in the near future – opening up the prospect of automatic x-ray scoring in real-word practice, without the need for routine involvement of a specialized radiologist.
While scoring of erosions has long been the ultimate hard endpoint of therapeutic efficacy in rheumatoid arthritis and psoriatic arthritis, it is rarely performed in practice outside of clinical trials. This is partially because it remains resource-intensive, but also because there remains significant inter-rater variation in assessment. While qualitative assessments of radiographic progression can often be made if historic radiographs are available, these fail when imaging across the course of disease is not co-located.
Assessment of hand radiographs therefore remains an Achilles’ heel amongst objective investigations. While the films may literally be black and white, their interpretation remains somewhat less easily defined. These difficulties have partially led to the reduced utilization of plain-film x-rays in real-world rheumatoid arthritis care, despite their obvious utility.
Of the practical applications of deep learning in rheumatology, detection of erosions on plain-film x-ray has always been a candidate for early application – a useful measure, yet tedious and inconsistent in its capture, and with raw data already in electronic form. This promise, to date, has not yet come to fruition.
Work presented at ACR Convergence 2020 seeks to embrace this potential. Investigators from Taoyuan, Taiwan, collaborating with an American biotechnology company, presented data regarding their artificial intelligence model to automatically score hand x-rays for erosions using the modified Sharp/van der Heijde score (abstract #0493).
Using 1397 x-rays from 450 rheumatoid arthritis patients, and a novel machine learning detection technique, they were able to develop an algorithm with comparable performance to a radiologist. The model, using a graph convolutional neural network, was able to detect the joint area and score the relevant joint space without supervision, and process the score in approximately one second. This performance extended across all joints relevant to rheumatoid arthritis in the hand and wrist.
While further validation might prove useful, this technology tantalizingly opens up a number of prospects. Not only could this reduce the burden on radiologists, it could pragmatically allow for a revival in erosion scoring in clinical practice, including in low-resource settings, and could ensure ready consistency of scoring within clinical trials. Other similar applications within different rheumatological diseases may also be future targets for similar development – living in the hope that we might be able to delegate imaging scoring to the computers, leaving us free to do so much more.
If you are a health practitioner, you may Login/Register to comment.
Due to the nature of these comment forums, only health practitioners are allowed to comment at this time.