AI in RA: the story grows along the patient pathway Save
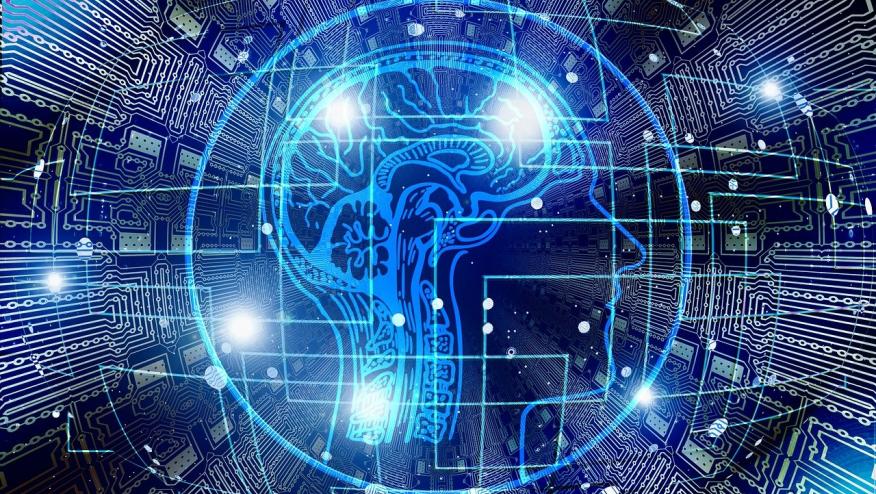
The use of artificial intelligence (AI) to analyse large data sets using its subset component machine learning (ML) has taken centre stage recently in the management of rheumatic conditions, including rheumatoid arthritis (RA). The use of AI is now starting to cross the various stages in patient care from detection, diagnosis, treatment and the prediction of long-term outcome. Combining large data sources from patient factors, comorbidities, diagnostics and radiology, data can be analysed with ML to provide prediction scores of outcomes such as the presence of RA, response to treatment and long-term outcome.
We have recently published our work using AI to predict the presence of inflammatory arthritis from analysing general practitioner (GP) referral letters (Wang et al. 2023). The use of this digital technology should reduce the administrative and time burden required to manually triage the referral letters and should also in the long-term improve the prediction of the presence of inflammatory arthritis.
At EULAR 2023, Fujii T et al in OP0190 looked at the use of ML in predicting the development from undifferentiated arthritis (UA) to rheumatoid arthritis (RA). A total of 322 UA patients in the KURAMA cohort were analysed. 24 clinical features, which are easy to obtain in daily clinical practice were used to train the model. The performance of the model was good with 73.5%, 74.2%, 74.5%, and 85.1% in precision and 0.760, 0.734, 0.748, and 0.895 in AUC for Random Forest (RF), XGBoost (XGB), Logistic Regression (LR), and Deep Neural Network (DNN) respectively with DNN showing the highest performance. The DNN model was applied to external validation data from ANSWER cohort and the prediction accuracy was 80.0%. This study shows that using parameters available in clinical practice, a DNN model that effectively predicted RA development in internal and external UA datasets can be utilised.
Another use of AI in predicting RA was shown in OP0002 by Li Y, where a deep learning AI-method that automatically analyses extremity MR scans by pre- and post-processing images, in order to predict RA at an early stage. MR scans of the hands and feet from a total of 1974 patients were collected, consisting of 1247 early onset arthritis (EAC) patients, of whom 538 developed RA in two years, and 727 clinically suspect arthralgia (CSA) patients, of whom 113 developed RA. Models trained separately on the wrists, MCPs and feet, received a mean AUC of 0.679, 0.647, 0.664, and 0.688, 0.669, 0.715, for the early onset arthritis (EAC) and clinically suspect arthritis (CSA) group, respectively. From 1,974 people analysed using this AI-method, 651 went on to develop RA. These accuracies were close to the expert-level using RAMRIS, with reported AUCs of 0.74 and 0.69 in predicting RA in CSA.
Applying a machine learning approach might enable identifying patients at high risk of RA progression and improve the clinical management of CSA and UA patients. As early diagnosis and treatment improves long-term outcomes in RA, using AI may be another tool that we can use to achieve this goal.
References
Bing Wang, Weizi Li, Anthony Bradlow, Eghosa Bazuaye, Antoni T.Y. Chan,
Improving triaging from primary care into secondary care using heterogeneous data-driven hybrid machine learning, Decision Support Systems, Volume 166, 2023, 113899.
If you are a health practitioner, you may Login/Register to comment.
Due to the nature of these comment forums, only health practitioners are allowed to comment at this time.