Better assessment and management of multimorbidity Save
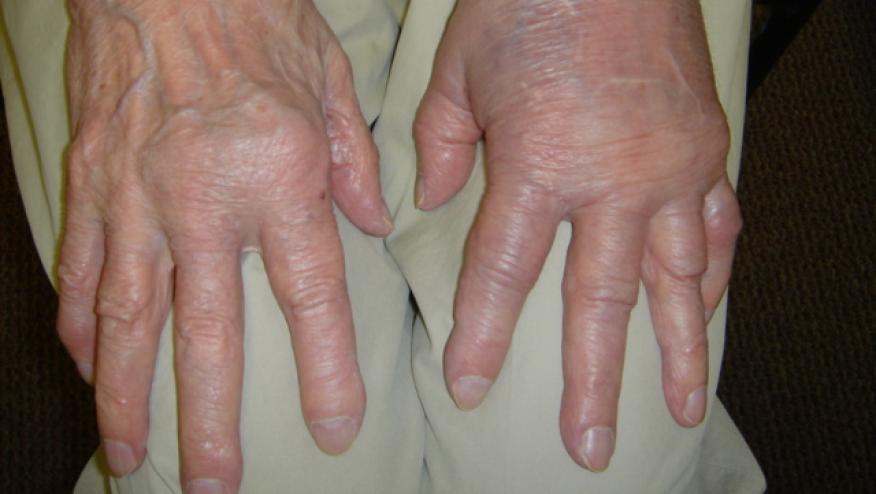
Multimorbidity, the presence of multiple overlapping chronic comorbid conditions, is increasingly common at first presentation of rheumatoid arthritis (RA), increasing as a person gets older and/or during disease progression. Appropriate assessment and management of comorbidities and multimorbidity in patients with RA is vital for overall health and well-being of the patient, as well as to encourage treatment adherence, and manage treatment side-effects, while addressing social, psychological and quality of life factors.
Results from the Canadian Early Arthritis Cohort (CATCH) (#0798) demonstrate the impact on disease activity in patients with multiple comorbidities. This study of over 2000 patients with early RA used the Rheumatic Disease Comorbidity Index (RDCI) to assess the influence of comorbidities on the trajectory of various components of disease activity, including tender and swollen joint counts, patient and physician global assessments, inflammatory markers and pain. The presence of multiple comorbidities was associated with worse improvement in disease activity in the first year of treatment. The effect was most particularly marked when assessing swollen joint count, and patient and physician global assessments. This brings home the impact of high burden of comorbidity at first presentation of RA.
Whilst tools such as RDCI are effective in assessing comorbidity status, work continues to find effective ways in which to incorporate this into clinical practice, given the huge impact of multimorbidity and comorbidity on patient experience of disease and overall outcomes. Two abstracts at ACR21 describe the use of machine learning approaches to identify comorbidity clusters which can be applied in clinical care.
B. England et al (#1924) constructed RA cohorts within the insurance database, MarketScan, and Veterans Health Administration, between 2006 and 2015. Forty-two chronic conditions were selected to form clusters within the cohort. A total of seven clusters (four in MarketScan, three in Veterans Health Administration) were identified and graded low, moderate or severe, with those in moderate/severe groups noted to be older, frequently male, and with greater comorbidity burden. Both cohorts featured a cluster characterised by mental health and chronic pain, associated with higher mortality.
A second abstract, by E. Atkinson et al (#1450) also applied machine learning to yield eight comorbidity clusters in a cohort of just over 1400 patients with RA. These clusters were characterised by anxiety and depression; cardiorespiratory conditions; vascular disease including hypertension, hyperlipidaemia and peripheral vascular disease; and obesity.
Both of these abstracts demonstrate possible ways to identify comorbidity clusters within patients with RA which can help to guide targeted assessment and management. Additionally, whilst the overlap of RA with mental health is well-established, these studies highlight the importance of considering these within comorbidity indices, especially given the impact on long-term outcomes and mortality. The abstracts on these topics at ACR21 serve to highlight an ongoing need for further research and improved management in this area, to ensure we are addressing all aspects of health and wellbeing in the care of RA patients.
If you are a health practitioner, you may Login/Register to comment.
Due to the nature of these comment forums, only health practitioners are allowed to comment at this time.