Predicting flares in rheumatic diseases with machine learning Save
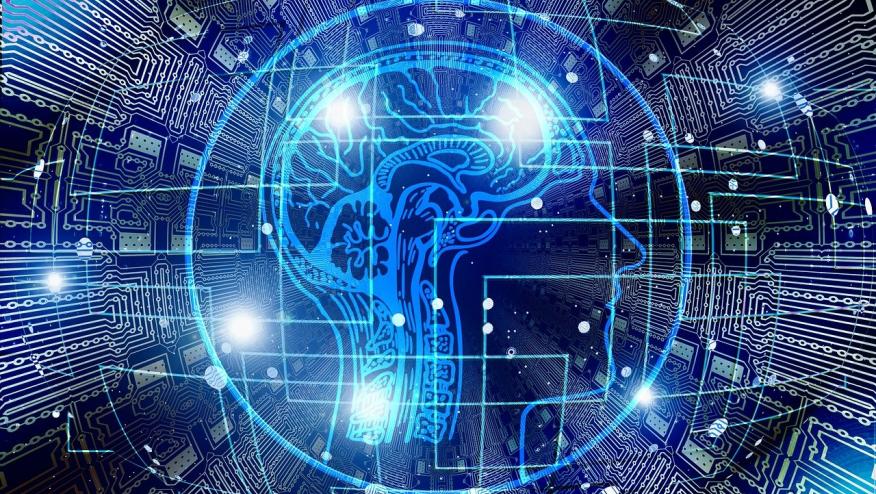
Rheumatic diseases, such as axial spondyloarthritis (axSpA) and rheumatoid arthritis (RA), are marked by unpredictable disease flares that adversely impact quality of life and long-term outcomes. Advances in machine learning (ML) provide a promising avenue for predicting these flares, enabling proactive management and personalised interventions. At ACR 2024, there were some abstracts that explored leveraging ML to predict flares in rheumatic diseases, their methodologies, and their implications for clinical practice.
Researchers used the international METEOR-SpA registry to develop ML models for predicting flares in patients with axSpA receiving biologic or targeted synthetic disease-modifying antirheumatic drugs (b/tsDMARDs). A flare was defined as an increase in the axSpA Disease Activity Score (ASDAS) or the Bath Ankylosing Spondylitis Disease Activity Index (BASDAI). The logistic regression model demonstrated strong performance, with an AUC-ROC of 0.708 and sensitivity of 76.5%. Predictors of flares included history of enthesitis and prior flares, duration of b/tsDMARD treatment and lower baseline ASDAS values. These insights enable clinicians to identify high-risk patients early and tailor treatment strategies to prevent flares (Abstract #0559).
A large-scale analysis at the Royal Berkshire NHS Foundation Trust, UK applied Long Short-Term Memory (LSTM) networks and neural networks (NN) to predict flares in RMD patients. The study utilised a dataset of over 140,000 patients, including electronic health records and patient-reported outcomes. LSTM models achieved an accuracy of 89.3% and an AUC-ROC of 0.76 for predicting flares six months before clinical visits. Predictive accuracy declined slightly when forecasting three months in advance, underscoring the challenge of shorter-term predictions. The models demonstrated potential for integration into clinical workflows, allowing patients and providers to anticipate flares and implement timely interventions, such as medication adjustments (Abstract #1722).
A collaborative effort between the National Health Service (NHS) in the UK and Princeton University developed an AI-based algorithm to enhance early detection and management of RA. A neural network achieved 87.6% accuracy in binary classifications of early inflammatory arthritis (EIA). The system predicted a 41.6% improvement in scheduling high-risk patient appointments. By incorporating serological data into flare risk predictions, this approach supports faster diagnosis and prioritisation of care, potentially improving long-term outcomes (Abstract #1744).
The ability of ML to identify flare predictors across multiple domains highlights its utility in rheumatology. By combining clinical, serological, and patient-reported data, these models enhance our understanding of disease trajectories and enable precision medicine.
However, challenges such as data quality, algorithm interpretability, and integration into clinical systems remain. As these models are refined and validated, they hold the potential to revolutionise patient care, reducing the burden of flares and improving quality of life. Future efforts should focus on addressing technical challenges and ensuring equitable implementation across healthcare systems
If you are a health practitioner, you may Login/Register to comment.
Due to the nature of these comment forums, only health practitioners are allowed to comment at this time.