Better at Predicting RA from MRI: Humans or Computers? Save
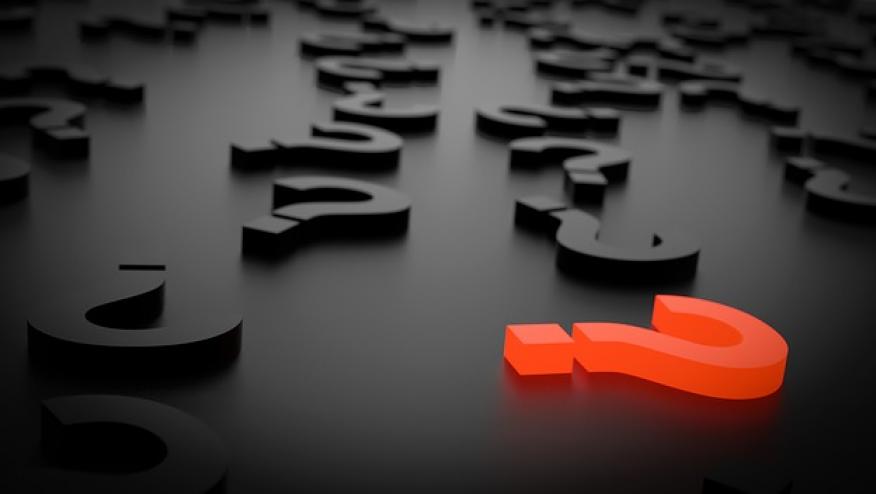
An artificial intelligence model to assess pre-RA hand MRIs has confirmed what human readers have known before: that bone marrow edema and tenosynovitis are objectively the best predictors of future RA development in patients with clinically suspect arthralgias or undifferentiated arthritis.
The computer cannot outperform human readers yet though, according to data presented in the opening abstract plenary at EULAR 2023 in Milan, Italy.
Early prediction of RA would help clinicians initiate effective therapies early, to better establish control within a therapeutic window of opportunity for long-term success in RA treatment, and perhaps, in the future, even prevent the development of RA altogether. In this population, usually with inflammatory arthralgias and either early synovitis or ACPA seropositivity, a number of features have previously been identified that predict the development of persistent RA, including bone marrow edema and tenosynovitis. RAMRIS, the Rheumatoid Arthritis Magnetic Resonance Imaging Score, is a score that captures some of this: it uses synovitis, bone marrow edema, and erosions at 23 joint sites of the dominant hand and wrist to create a score as a therapy outcome, derived through an OMERACT expert consensus process.
However, the rise of artificial intelligence in radiology has now turned to RA prediction – particularly deep learning, which can best analyse patterns within medical imaging. Plausibly, it could help to determine whether sophisticated combinations of findings on MRI, and novel features which might not ordinarily be adequately captured by traditional radiological descriptions, might be key to determining whether patients will develop persistent RA. While they could be used to try and outperform human algorithms by looking for these novel features, they could also help to confirm the performance of existing approaches like RAMRIS. Furthermore, if explainable techniques are used when developing the deep learning tools, areas contributory to that decision can be identified for better use in clinical care.
Data from a radiology-rheumatology collaboration presented on Wednesday’s opening abstract plenary at EULAR 2023 might shed more light in this way (OP0002).
Investigators from Leiden, the home of a long-established large early arthritis cohort, looked at the performance of a deep learning artificial intelligence model and compared it to a RAMRIS cut-off. In their well-curated clinical cohort of 1974 pre-RA patients, including 1247 with early onset arthritis and 727 with clinically suspect arthralgia, approximately one-third – 651 patients – went on to develop persistent RA. Using typical techniques and these data, but no MRIs from healthy controls, the investigators built a deep learning model to predict those who would develop RA, and identify contributory areas on imaging.
Using MRIs of the wrist, and MCP joints, this algorithm was able to achieve reasonable accuracy, with an AUC of 0.68 in the early onset arthritis group, and 0.73 in the clinically suspect arthralgias group. Looking at wrists, MCPs, or feet, in combination only led to minor incremental benefits over looking at any of the three individual components alone. RAMRIS, the human-derived model, performed similarly to this algorithm, with an AUC of 0.74 in early onset arthritis, and 0.69 in clinically suspect arthralgias. Critically, the deep learning algorithm identified areas culpable corresponding to what had already been identified using conventional techniques – bone marrow edema and tenosynovitis.
Nevertheless, both the artificial intelligence and human algorithms had imperfect performance, and greater prediction accuracy may be needed in the future to stratify patients for intervention. The authors speculated that incorporating data from healthy controls may improve algorithm performance, but it is clear this may also not be sufficient to allow it to be accurate enough as desired. While both imaging and deep learning techniques are likely to improve in the future, it is possible that MRI alone can never be able to completely predict who will go on to develop RA. It is also certainly possible that combining different modalities of data – such as imaging, cytometry, transcriptomics, and patient-reported outcomes – may well be necessary to achieve optimal predictions.
Multi-modal biobanks, capturing all of these data, are key to understanding the development of RA better as our speciality works towards better early treatments and perhaps future prediction.
If you are a health practitioner, you may Login/Register to comment.
Due to the nature of these comment forums, only health practitioners are allowed to comment at this time.