AI meets Rheumatology: ChatGPT and patient response optimization Save
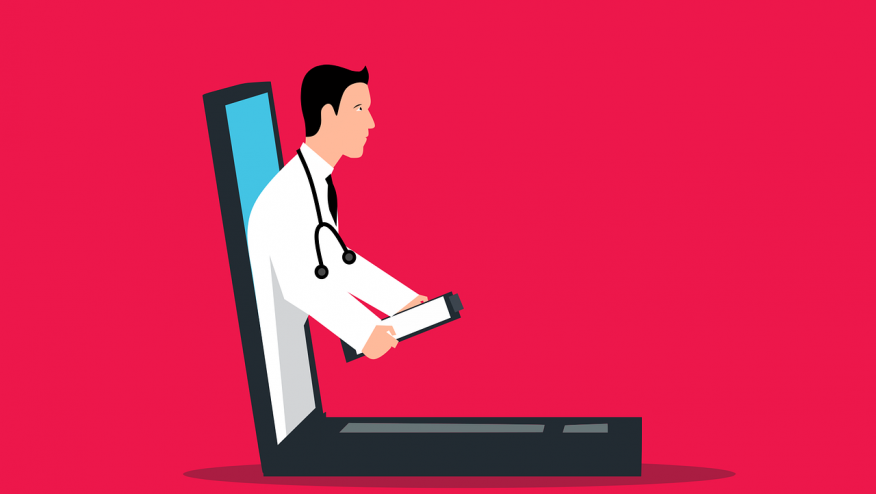
Large language models, such as ChatGPT, are advanced systems trained on vast amounts of text data, far exceeding what a human can read in a lifetime, to understand and generate human-like language. With these tools at our disposal, they are inevitably making their way into healthcare.
One notable example is response optimization for SLE (systemic lupus erythematosus) patients, as highlighted in abstract #0989 at #EULAR2024. Large language models have democratized access to sophisticated linguistic tools, allowing non-computer scientists to utilize them through simple prompts—commands in everyday English.
In this study, researchers compiled 95 frequently asked questions regarding SLE and gathered responses from five rheumatologists, which they then compared to responses generated by ChatGPT-4. These responses were evaluated across six domains (each scored from 0-10) by independent rheumatologists and patients. The process was blinded to eliminate bias.
According to the independent rheumatologists, ChatGPT outperformed human experts across all six domains: scientific validity, logical consistency, comprehensibility, completeness, satisfaction level, and empathy. From the patients' perspective, there was no significant difference between the responses from ChatGPT and the rheumatologists. Importantly, no safety issues were identified.
While concerns about hallucinations and ethical issues persist, rheumatologists must recognize the evolving landscape. In my view, large language models represent a significant advancement, akin to the introduction of electronic medical records. With numerous tech companies and EMR systems like Epic rolling out early versions of patient response portals, we will likely see this technology become mainstream in the coming years. Notably, these models are continually improving—earlier versions of ChatGPT exhibited more frequent hallucinations in response to rheumatology questions compared to the current iteration. Significant fine-tuning is occurring behind the scenes.
This study, among others, demonstrates that large language models enhance patient education by generating understandable explanations of medical conditions and treatments. Overall, the integration of large language models into rheumatology promises improved patient outcomes and more efficient clinical workflows.
If you are a health practitioner, you may Login/Register to comment.
Due to the nature of these comment forums, only health practitioners are allowed to comment at this time.