Artificial intelligence brings clarity to early bony changes in inflammatory arthritis Save
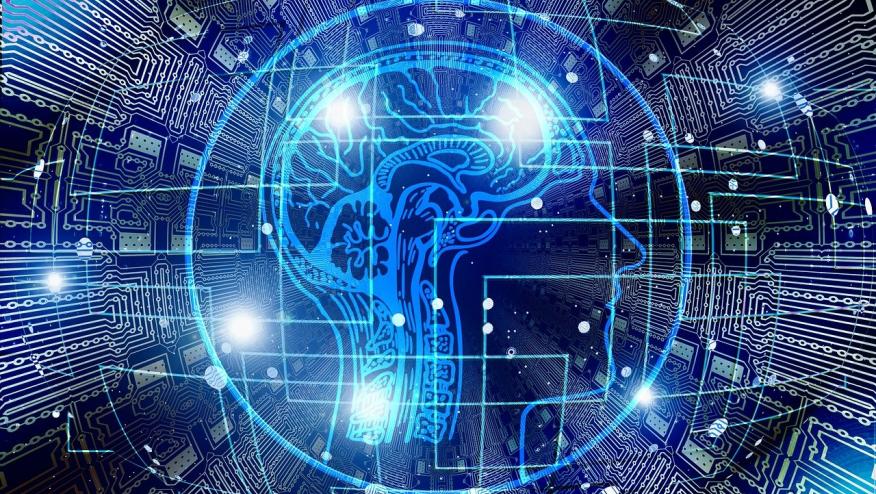
Subtle early bony changes in inflammatory arthritis can be hard even for experienced rheumatologists to interpret, even though they have traditionally been considered to become disease-defining as they progress. As computational techniques have become more sophisticated and more accessible, questions have been asked as to whether such technology can be harnessed to identify inflammatory arthritis more rapidly.
Lukas Folle and colleagues from Friedrich-Alexander-Universität in Erlangen-Nuremberg, Germany presented work at the EULAR 2021 Virtual Congress addressing this, in a collaboration between rheumatologists and computer scientists. They used artificial intelligence methods to analyze imaging of the hand, with the aim of identifying patients different inflammatory arthritis from healthy controls, purely on the basis of analysis of the bony surfaces of a single joint – specifically, the second metacarpophalangeal joint.
To do this, they used high-resolution peripheral quantitative computer tomography (HR-pQCT) scans, which are relatively accessible but characterize bone well. HR-pQCT scans are most commonly used to non-invasively capture three-dimensional bone architecture in diseases like osteoporosis, but can be used to understand subtle changes in the bone around this joint. Importantly, few of the patients in this study had erosions, so this study was reliant on picking up subtle changes to the bone that might precede erosions.
To do this, they developed an algorithm using a popular method in machine learning called convolutional neural networks, which mimics neural networks in the brain in processing variable length sequences of inputs. They used this to analyze scans from the university’s clinic, taken of patients with seropositive rheumatoid arthritis and psoriatic arthritis, and compared them to healthy controls. After testing and validating this algorithm, they tested it on further patients from the clinic to see how well it performed.
Encouragingly, Lukas Folle and his colleagues found that the algorithm was able to identify a patient’s disease correctly 89% of the time, purely on the basis of the scan of the bone around the second metacarpophalangeal joint, with an area under the curve of 91% indicating excellent analytical performance of the algorithm.
The computational approach of this algorithm tellingly allowed the investigators to demonstrate which regions of bone were important. The algorithm was able to show researchers that areas of high curvature were the most telling, in keeping with other theories about microstructural changes at the second metacarpophalangeal joint in both rheumatoid and psoriatic arthritis.
"AI is at its most useful when it's being used not to automate human tasks, but to break new scientific ground and develop new knowledge," said Dr. Chris McMaster, a final year rheumatology fellow and clinician data scientist from Melbourne, Australia. "This is a very exciting use of state-of-the-art algorithms that could potentially lead to earlier diagnosis and better outcomes for patients with inflammatory arthritis."
While the investigators hope to use this to find more about which regions of bone surface are most relevant to bony changes in inflammatory arthritis, this approach holds enormous promise for improving diagnosis of both rheumatoid arthritis and psoriatic arthritis. Potentially scalable with minimal human input, such technology could be used to hasten a provisional diagnosis of inflammatory arthritis in primary care, and identify such patients to rheumatologists more rapidly.
‘Differential diagnosis of RA and PsA using neural networks on three-dimensional bone shape of finger joints’, EULAR Virtual Congress 2021. https://ard.bmj.com/content/80/Suppl_1/86.abstract
Folle, L., Meinderink, T., Simon, D. et al. Deep learning methods allow fully automated segmentation of metacarpal bones to quantify volumetric bone mineral density. Sci Rep 11, 9697 (2021). https://doi.org/10.1038/s41598-021-89111-9
If you are a health practitioner, you may Login/Register to comment.
Due to the nature of these comment forums, only health practitioners are allowed to comment at this time.