Can Neural Networks Answer Important Diagnostic Dilemmas in Rheumatology? Save
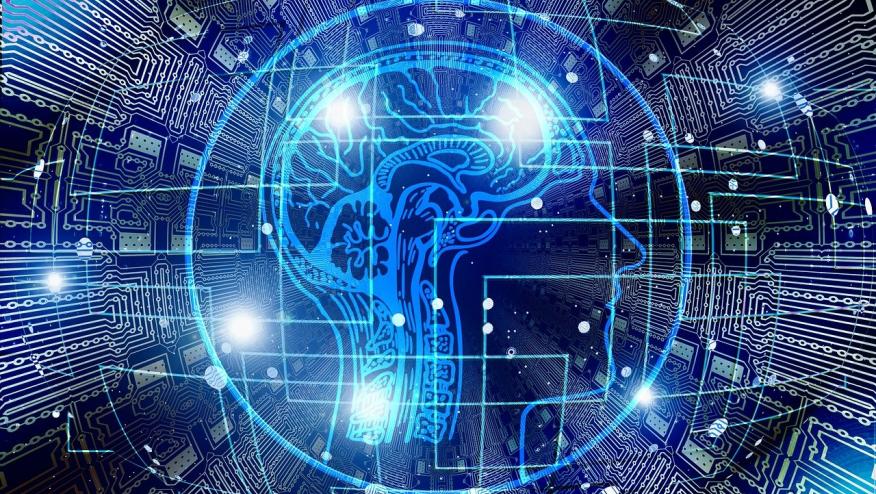
A clinician faces several vexing diagnostic dilemmas, multiple times during a busy time in the clinic. Is this Seropositive RA with psoriasis or psoriatic arthritis (PsA) with a marginal rheumatoid factor? Is this PsA sine psoriasis (or minimal psoriasis often hidden from plain sight) vs seronegative RA?
In the absence of diagnostic serologic tests or biomarkers, the diagnosis often depends on the clinical acumen of the physician. It is not uncommon for a patient to receive multiple conflicting diagnoses, leading to patient confusion, mistrust and lack of adherence to medical treatments.
Imaging studies can be helpful in differentiating between the different inflammatory arthritides. However, there is significant overlap and, more often than not, they are not read by subspecialty trained musculoskeletal radiologists.
A neural network is a series of algorithms that endeavors to recognize underlying relationships in a set of data through a process that mimics the way the human brain operates. In this sense, neural networks refer to systems of neurons, either organic or artificial in nature.
Neural networks can help computers make intelligent decisions with limited human assistance. This is because they can learn and model the relationships between input and output data that are nonlinear and complex.
An interesting study (Abstract #242) was presented in the 2022 American College of Rheumatology (ACR) Convergence. The investigators wanted to establish whether neural networks can distinguish seropositive rheumatoid arthritis (RA+), seronegative RA (RA-), and psoriatic arthritis (PsA) using hand MRI data based on the structural inflammation patterns.
They utilized neural networks based on the ResNet architecture that were trained to distinguish (i) RA+ vs. PsA, (ii) RA- vs. PsA and (iii) RA+ vs. RA- using hand MRI data. MR sequences consisted of five different sequences (T1 coronal, T2 coronal, T1 coronal and axial fat suppressed contrast-enhanced (CE), and T2 fat suppressed axial). Two neural network trainings were performed, where (i) the neural network had access only to MR data and (ii) then to MR and clinical and demographic data. Using a specialized visualization technique for the neural networks (occlusion) the regions most important for the network were recorded and correlated to the respective anatomical regions by an experienced rheumatologist.
Hand MRI scans from 502 patients (135 RA-, 190 RA+, 177 PsA) were assessed. Differentiation between disease entities as measured by the AUROC was 75% (SD 3%) for RA+ vs PsA, 74% (SD 8%) for RA- vs PsA, and 67% (6%) for RA+ vs RA-. All MR sequences were important for neural network performance with marginal differences in performance, with the caveat that no contrast-enhanced sequences were utilized for that study. Adding demographic and clinical parameters to MR data did not improve the performance of the neural network significantly, although the data regions important for deep learning had also shown clinical relevance.
The investigators concluded that neural networks can help distinguish between different forms of inflammatory arthritis based on MRI inflammatory patterns, apparently focusing on regions of clinical relevance.
This study needs to be validated in a larger population and, possibly, also examine contrast-enhanced sequences. If sequences and regions become standardized, this could evolve into a useful tool that can enhance the differential diagnostic ability of clinicians and MRI radiologists, potentially decreasing the lag between initiation of symptoms, diagnosis and onset of treatment, thereby improving the quality of life of patients and preventing radiographic progressions, damage and, potentially, disability.
If you are a health practitioner, you may Login/Register to comment.
Due to the nature of these comment forums, only health practitioners are allowed to comment at this time.