ICYMI: Taking AI Out of AutoImmunity: Predicting disease before it develops Save
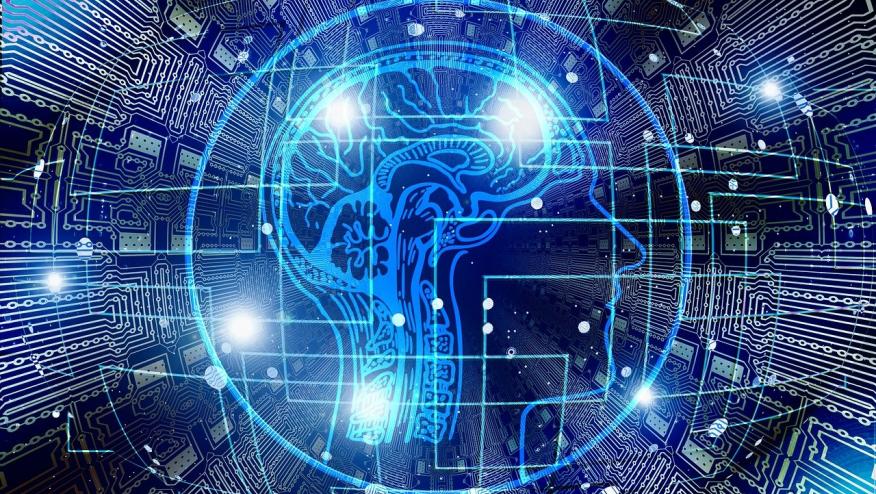
Autoantibodies play a crucial role in diagnosing and classifying autoimmune and connective tissue disorders. However, the predictive value of a positive antinuclear antibody (ANA) test—especially in the absence of other clinical symptoms—remains a challenge. A positive test often leads to further testing, yet it does not necessarily indicate whether a patient truly has an underlying autoimmune disease. The development of artificial intelligence (AI) and machine learning (ML) algorithms presents an opportunity to interpret autoantibody tests and predict autoimmune diseases.
In recent years, there has been an increased demand for autoantibody testing. This has placed greater pressure on laboratories to interpret results accurately and efficiently.
A notable approach presented in Late Breaking Abstract L11 employed AI-based algorithms to enhance ANA interpretation. Researchers evaluated eight ML models, and four convolutional neural networks designed to classify and distinguish ANA patterns. This study showed that several of these algorithms could nearly match the accuracy of trained laboratory interpreters.
When ANA results return positive, the question arises: who is truly at risk of developing autoimmunity?
Abstract 1526 tackled this by leveraging electronic health record (EHR) data from the TriNetX database. Researchers identified clinical variables associated with positive ANA tests, such as diagnostic codes, medications, and prior lab results. By training an ML model on these parameters, they were able to stratify patients based on their likelihood of developing systemic lupus erythematosus (SLE). The study found that patients with higher model scores had a significantly increased risk, highlighting the role of AI to support clinicians in identifying individuals who may benefit from closer monitoring.
The development of predictive models requires robust validation across diverse populations.
In Abstract 0843, researchers used data from the Nurses’ Health Study to create three predictive models based on (1) genetics, (2) environmental & lifestyle factors, and (3) a combination of both. When these models were applied to an external population, the combined genetic-environmental model showed the highest accuracy in predicting which individuals were likely to develop SLE. This highlights the importance of integrating multiple risk factors to improve prediction accuracy.
As evidenced by these abstracts, artificial intelligence and machine learning models have enormous potential to 1) accurately and efficiently interpret positive autoantibody tests and 2) predict the risk of autoimmunity. While the algorithms can perform sophisticated functions, the output relies on the input, specifically the quality and diversity of the trained data. Application of these algorithms to external cohorts is critical for validation, which is a necessary step prior to implementation into real-world clinical practice. By identifying individuals at risk for autoimmunity, preventive measures can be taken to minimize the risk.
If you are a health practitioner, you may Login/Register to comment.
Due to the nature of these comment forums, only health practitioners are allowed to comment at this time.